Sam is an astronaut who has been on the Moon for almost three years, accompanied by GERTY, a robot capable of making autonomous decisions regarding his physical and mental health, prioritising his well-being (it seems quite promising, don’t you think?). The film Moon introduced a slightly less catastrophic perspective than Stanley Kubrick’s classic 2001: Space Odyssey and his robot HAL 9000, but it did not completely disregard the “recipe” created by Hollywood – an artificial intelligence (AI) solution that seems to solve our problems and ends up turning against us. The GERTY and HAL 9000 robots, which provided essential support in monitoring people’s health status and making autonomous (medical) decisions, are just two of many fictional examples that have contributed to undermining our confidence in AI.
The journey that separates fiction from reality is long and still characterised by doubts. What role can robots and AI systems play in healthcare? From diagnostic tools to decision support, as well as more precise treatment plans or personalised therapies, the answers to medical challenges are multiplying, in Portugal and in the world.
Accurate diagnostics? AI can help!
An IDC study commissioned by Microsoft revealed that 79% of healthcare organisations already resort to AI. It is estimated that by 2025, there will be a 60% increase in the adoption of AI-based solutions by healthcare providers. A 2020 MedTech Europe study indicated that using AI in healthcare could save around 400,000 lives annually, and approximately €200 billion in Europe. A study from the University of California, Los Angeles (UCLA) showed that an AI tool detected prostate cancer with an accuracy of 84%, while doctors showed a rate of 67%. AI demonstrated high sensitivity on ignored or incorrectly annotated indicators on chest X-rays. When looking at these statistics, it is not surprising that INESC TEC is working on multiple solutions where AI plays a crucial role, like the European PHASE IV AI (diagnosis, treatment and data management in critical areas like cancer), AI4Lungs (early and accurate diagnosis of lung diseases), LUCCA (identification of genetic and molecular patterns associated with lung cancer), CINDERELLA (prediction of aesthetic results of reconstructive surgeries in breast cancer patients) and the CADPath.AI (an AI prototype that allows optimising the diagnosis of colorectal pathologies) projects. The goal? Promote more accurate diagnoses and treatments.
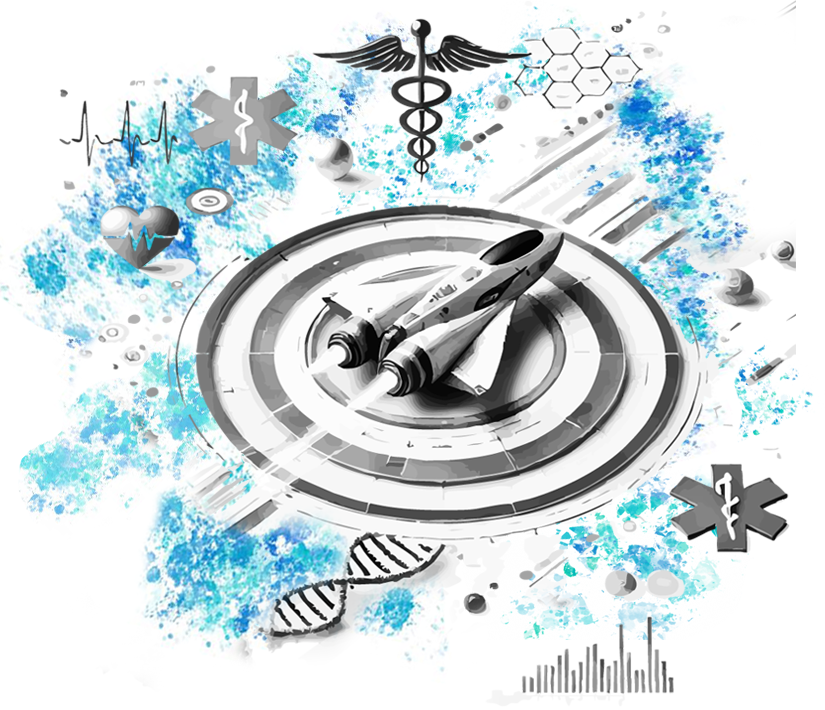
But when we talk about AI applied to healthcare, it must be explainable and ethical. Who is responsible when an algorithm fails? How do we build trust between physicians, patients, and AI systems? Can the use of biased systems lead to wrong decisions or perpetuate inequalities?
Tiago Gonçalves, researcher at INESC TEC, started working with AI intelligence in health, namely in projects related to medical image analysis, such as CINDERELLA. “Twenty years ago, the priority was to cure the disease, and little thought was given to the aesthetic impact. Today, especially in the case of breast cancer, people are more concerned about this aspect. Therefore, this work does not focus on diagnosis or treatment, but on the quality of life of patients after procedures. Our goal is to create algorithms that help assess whether the aesthetic result was satisfactory, using photographs taken before and after surgery.” We are talking about algorithms that analyse asymmetries, scars and other visible changes in images.
Now let’s think about an algorithm that not only classifies the images, but also presents a “salience map”, which highlights the most relevant areas of the image for the AI decision and adds textual explanations. INESC TEC researcher Isabel Rio-Torto explained everything: “for example, if a lung collapsed, it makes sense that the map shows the area of that collapsed lung. Or in the case of skin lesions, it will be beneficial for the map to highlight areas with more irregular edges, something that may be indicative of cancer. The idea is using an algorithm that classifies X-ray images, creates a visual map, and generates a text explanation.” The benefits? “A recent article showed that radiologists prefer to receive textual and visual explanations together. They measured the difference between providing only visual maps, only textual explanations, or both, and concluded that the combination is the preferred option”. But will this explanation be enough?
Tackling bias and inequity: data quality matters
According to Tiago, the use of AI in high-stake decisions requires special care, since said decisions can have a direct impact on someone’s life and health status. Although algorithms are increasingly accurate, it is essential to test their limitations and adapt them to different realities, as protocols and contexts can vary greatly, e.g., from country to country. Algorithms developed in Europe may not work well in Africa, due to genetic, cultural and socio-economic differences.
A clear example of bias is the difference in the accuracy of medical sensors in people with different skin tones. “Oximetry sensors, for example, work better in people with light skin than in people with darker skin. This can lead to late or inaccurate diagnoses, simply because the data acquired do not reflect the reality of these populations. In other words, the problem begins with data collection and affects the entire process,” explained the researcher.
Regarding equity in AI, Tiago Gonçalves said that it is possible to adjust the algorithms to avoid discrimination. “Algorithms tend to benefit more common cases, because most available data focus on this reality. But we can incorporate mechanisms that recognise when a case is less common and adjust decisions to ensure greater accuracy.”
Isabel added that one of the issues of applying AI in clinical contexts is that, in most cases, the algorithms are trained with data from only one hospital. What about when we transition the same algorithm to another hospital? “For the same diseases, the results may not be so good. Simply change the equipment that took the radiograph or the light conditions, and it may affect the performance of the model. This is a problem that is not yet completely solved”.
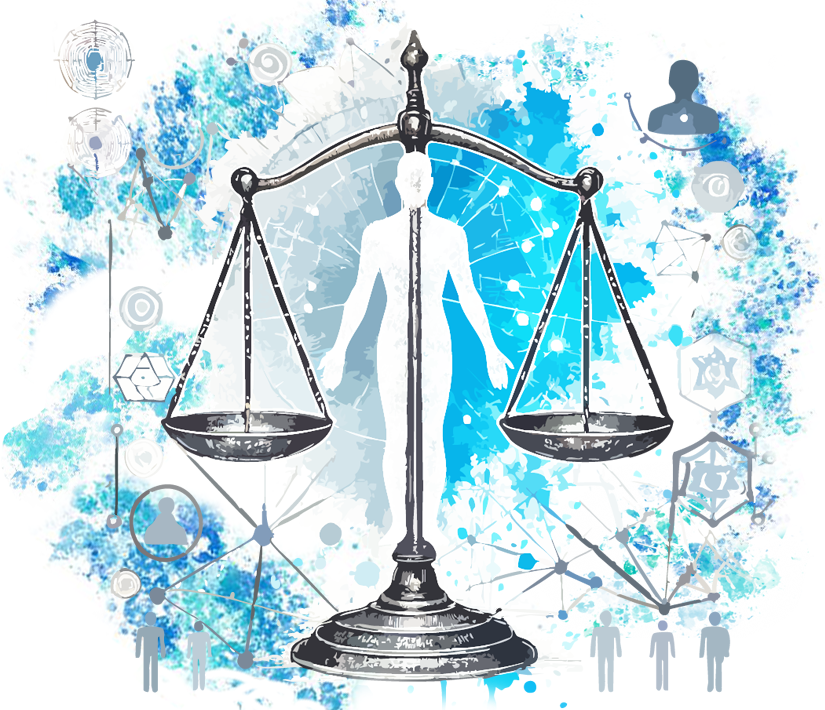
Effectively, working with quality data can prevent bias. “Algorithms learn from the data they receive. If they are trained with reports of doctors who make mistakes or have biased practices, this is what they will reproduce. It is known as ‘garbage in, garbage out’,” said Isabel Rio-Torto, mentioning the case of the COMPAS (Correctional Offender Management Profiling for Alternative Sanctions) system used in the courts of the United States, which demonstrated bias against the black population because the data used for training already reflected this inequality. “Algorithms totally depend on the data with which they are trained. If the data has noise, errors, or biases, the algorithms will learn that too. For example, in the case of rare diseases, where there is less data available, algorithms may not be as effective,” added Tiago.
Explainability and federated learning
The growth of explainable and interpretable AI was, according to Tiago Gonçalves, driven by these ethical issues. The motivation behind many advances in explainable AI is to ensure that systems are fair and transparent, especially in areas like healthcare.
But Isabel also mentioned the difficulty in explaining algorithms and ensuring that the models are, in fact, interpretable. “There are techniques that allow us to generate explanations a posteriori, but other colleagues and I are sceptical of these approaches. We have no assurance that these explanations match what the model is doing. The only way to ensure interpretability is to build the model with integrated explainability from the beginning.”
There is a long way to go to ensure these solutions are effective. The European Union’s AI ACT is a first step, but clear regulations that set standards for the development and application of AI in health are essential for Tiago. “Algorithms need to be monitored continuously, because reality changes. An algorithm that worked 10 years ago may no longer be adequate today.” One of the solutions being explored is federated learning, an approach that allows training models collaboratively without the need to centralise data while preserving data privacy. “Each hospital preserve their data locally but contributes to the development of a more robust global model,” clarified the researcher.
Tiago and Isabel agree when it comes to this: AI should always be perceived as a support tool, and not as a substitute for professionals. “The final decision must always be human. For example, radiologists have already admitted that AI is very useful for filtering the simplest cases, so that they can focus on the more complex ones. Therefore, AI is an ally, not a threat”, said Tiago. Isabel mentioned that it is unlikely that AI will do the work of a doctor, but it is essential that they understand their potential: “the final decision must remain with the doctor. I think that the new generation of professionals, who are already more accustomed to the technologies, will adopt these tools more easily, especially if they are introduced during training. Moreover, they can help doctors to better understand how algorithms work and support the training of doctors themselves.”
This also includes the need for collaboration between different areas of knowledge. “Researchers need to be aware of the impact of what they are developing, and healthcare, law, and policy professionals also need to understand the limitations and potential of AI. Only with this multidisciplinary collaboration can we ensure that technology improves people’s lives”, stated Tiago.
The success of AI models in the diagnosis and treatment of epilepsy
Did you know that AI can predict epileptic seizures with up to 99% accuracy? Let’s talk about real cases, where multidisciplinary collaboration already bears fruit. At the Neurology Service of the Centro Hospitalar Universitário São João (CHUSJ), the goal is to use a clinical decision support tool to help physicians in cases of refractory epilepsy (i.e., those whose epileptic seizures are not controlled with medications), namely in the interpretation of brain signals. José Almeida is the INESC TEC researcher who is developing this method of identifying epileptogenic regions using machine learning. “From recordings of intracerebral electroencephalogram (EEG) signals, we can extract elements like connectivity between different channels, applying algorithms to classify the areas of the brain that generate the seizures. Therefore, it is a software that makes use of different signals and brain images acquired during and between seizures, with high sensitivity”, he said.
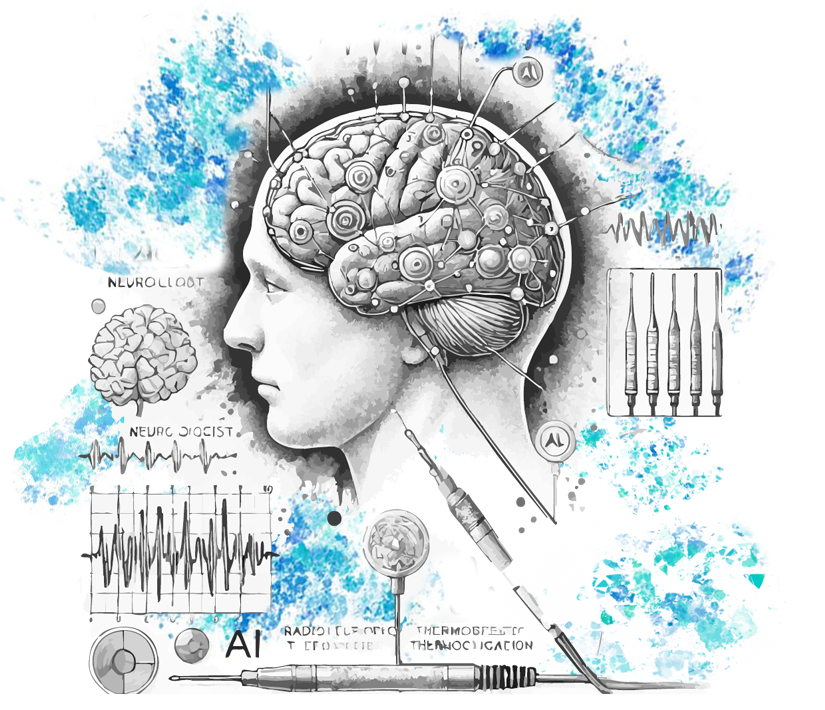
But José aims to go even further and is now working on a protocol that allows neurosurgeons to use the information extracted by the algorithms to define a treatment. We are talking about a technique called thermocoagulation that uses the intracranial electrodes of the EEG to emit radiofrequency impulses and increase the temperature in the selected region. The increase in temperature causes thermocoagulation, i.e., it burns the tissue in the target region, reducing or eliminating epileptic seizures. “What makes this research ground-breaking is the proposal to create a protocol that helps neurosurgeons apply the lesion more accurately, minimising side effects and improving treatment outcomes. Currently, this decision is made only by visual evaluation, which can be subjective and lead to errors, especially among less experienced teams,” explained the researcher. In addition to developing the protocol for the application of thermocoagulation, the research group intends to create tools that help assess the effectiveness of the procedure.
José Almeida pointed out that, although thermocoagulation is a relatively accessible, safe and simple procedure to implement – requiring only electrodes and a radio frequency machine –, there are still many challenges, like the liaison between hospitals to obtain patient data and the analysis of brain signals, which have very low amplitude.
One of the next steps is to combine these tools with other technologies for broader applications. “There is the possibility of creating implantable devices that address specific biomarker-based stimuli, such as those used in deep brain stimulation (DBS). The technology can also be adapted to treat other diseases,” he claimed.
In the real world, we are working on solutions that excel in collaboration between healthcare professionals and researchers and that seek to use AI ethically. So don’t worry; we are still a long way from finding the GERTY robot at the next medical appointment. But… until when?